Assessing the credibility of a crowd forecast
By Adam Siegel on April 20, 2022
"Yes, but who are these people?"
"What is their track record? Are they any good?"
"Why should I trust this?"
With increased attention to crowdsourced generated forecasts as guidance to
critical decisions comes the need to be as transparent as possible about the
credibility and trustworthiness of a current forecast. Am I looking at a forecast from only 5
people? Are the people who are participating in this question all new forecasters
with very little track record? Are they, on average, worse or better than the
median forecaster in the overall pool of participants?
We've always had this data available, but reserved it for curated reporting. Now we've introduced a new section on every question called "Forecast Profile." This is what v1 looks like:
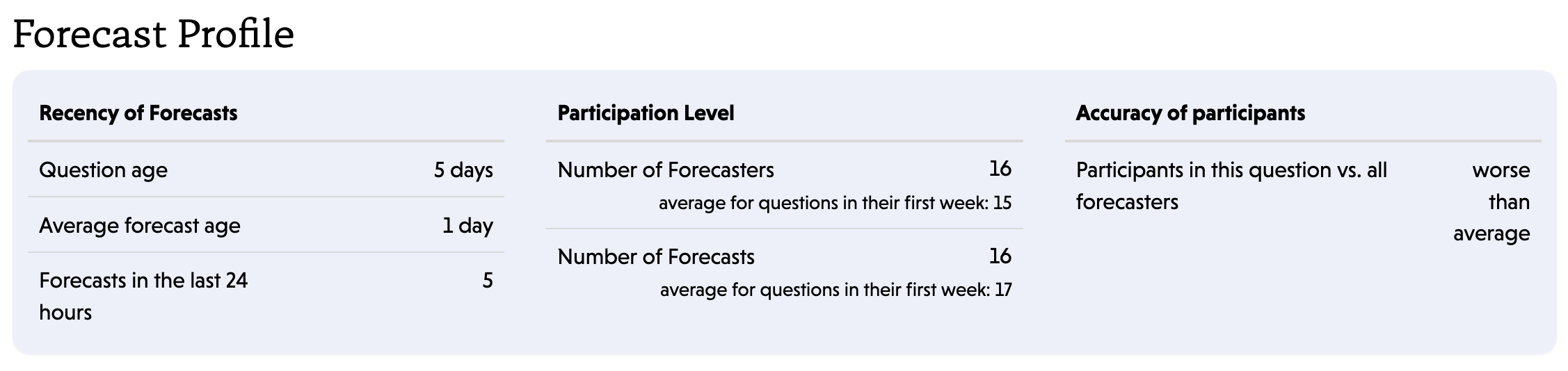
We're covering three major aspects of the crowd forecast here:
- Recency of Forecasts: How long has the question been running, and how recent are the updates? In some scenarios, it's important that the average forecast age is close to current. A short term question with lots of changing variables that could impact the outcome is most trustworthy with assessments using the most up to date information. A longer term question, however, that is much more static may be ok with an average forecast age that is older.
-
Participation level: How big is the participating forecaster pool, and how does that typically compare to questions on this particular site? Questions with a below average number of forecasters may be more technical, or lacking information and data to react to.
-
Accuracy of participants: Are the forecasters participating in this question more or less accurate than the pools of forecasters participating on any other question? Right now we give a simple assessment, but shortly you'll also be able to hover over that value and see the distribution of these forecasters.
As you can see, there's a lot of nuance here to consider. When we first began thinking about our approach, we considered rolling this all up into some sort of aggregated "trust score." We ultimately decided that would be too opaque and give a false sense of confidence in the forecast. A key lesson we've learned over the years is not only do people care if you're directionally correct and well calibrated in your forecasts, but that they can trust us enough to leverage the information we're providing to help form their own judgments and analysis.
We have more ideas to continue to expand on this concept but we hope this is a first step in helping analysts and decision-makers alike further interpret the results they're looking at.