Gaming Prediction Markets
By Ben Golden on August 20, 2015
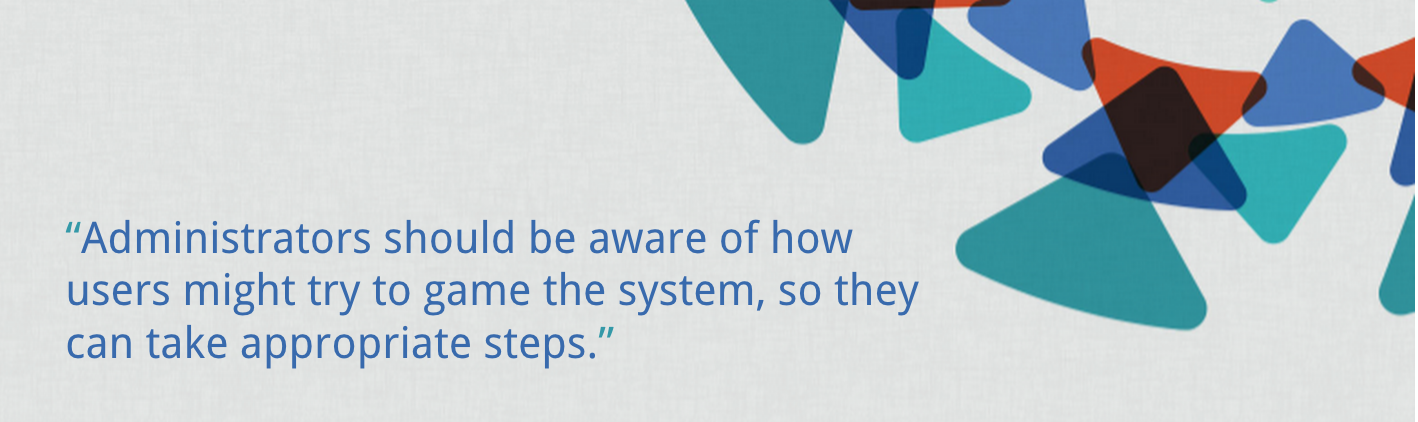
- Poorly formulated questions
At Inkling Markets, forecasters can propose their own questions, and can do so in ways that make it easier for them to profit. One way to do so is by setting initial forecasts inappropriately. If you set a question to start with a 50% likelihood when you actually think it should be 90%, you can gain points by correcting your own mistake.
-
Get in first
Similarly, users can correct obvious initialization errors made by others. This does improve the market forecast, but it's an improvement another user would have made anyway (assuming it's actually obvious). Users that get to questions first often do very well, even if they're not particularly good forecasters.
-
Aftercasting
Sometimes the result of a question is already known but it remains open, and users continue to "forecast" it. This tactic is highly profitable and essentially risk-free, and while it makes markets appear marginally more accurate at the end, it doesn't add any actual predictive value, since the result is already known.
-
Point dumping
This practice involves multiple users (or a single user controlling multiple accounts) forecasting in a way that intentionally benefits some users at the expense of others, and is explicitly banned by most prediction markets. It typically happens in free prediction markets where it's easy to create a new account, and in situations where user rewards are skewed toward the top. For instance, if a prediction market pays rewards its top five users, then it makes sense for the sixth and seventh place users to conspire to boost one of them into the top five, even if the other user suffers.
-
High-ish frequency trading
Users who monitor questions closely can revert forecasts when new or uninformed users forecast far outside an established consensus. As with getting in first, these corrections do improve forecast accuracy, but the reward is claimed by the first user to notice the change, and not by the most accurate forecasters. This issue also occurs in financial markets.
-
Bet long-shots
Inkling uses a scoring rule called LMSR, which allows users opportunities to earn incredibly large rewards when correcting relatively small errors near the extremes of a probability distribution. As an example, consider two forecasts:
- Type 1: a user adjusts the market forecast from 0.1% to 5%, when 5% is indeed the correct forecast
- Type 2: a user adjusts the market forecast from 40% to 60%, when 60% is the correct forecast
By my calculation, type 1 rewards its user 80% more, whereas type 2 has a 15 times larger effect on the market's accuracy, as measured by its Brier Score.
Conclusion
Some of these add no predictive value to the market, while others just make it difficult to discern who the most valuable market participants are. Mostly, prediction market administrators should be aware of how users might try to game the system, so they can take appropriate steps to limit these behaviors.
If you liked this post, follow us on Twitter at @cultivatelabs.
Ben Golden/@BenGoldn is an Engineer at Cultivate Labs