Playing with ChatGPT: Summarization of Forecast Rationales
By Adam Siegel on April 18, 2023
I've been asked quite a bit the past few months about the impact large AI Language Models will have on crowdsourced forecasting. Will you one day be able to type in a predictive query and be given some sort of probabilistic response? I'm sure that will happen in some form or another in the future. In the meantime, we're thinking about how tools like ChatGPT can be used to augment either the experience of forecasting or the analysis of forecasts.
I'll write about ChatGPT as a forecasting aid another time, but we've most recently been playing around with ChatGPT's text summarization capabilities. I've written a lot about the unsung value of the crowd forecasting process in our platform being the collection of qualitative rationales alongside people's probabilistic forecasts. In most of our sites we require people to enter these rationales because those who want to leverage the forecasts find them so valuable as context for the probabilistic trends and distributions.
Because of this, we spend quite a bit of time manually summarizing rationales for our reporting to our clients. Synthesizing the rationales in support of different viewpoints is one of the most important post-processing jobs we do. However, it has issues. It's time consuming, we can unintentionally introduce bias depending on the language we use to summarize the rationales, and providing updates, say on a weekly basis, means repeating the resource-intensive process all over again.
Manual and time consuming sound like a perfect use case for some automated technology which is why we were so interested in what ChatGPT could do. Ultimately our goal would be to do the same thing a human was doing: summarize the rationales representing different probabilistic judgments.
We started with the following prompt to ChatGPT using a question from INFER: "The following is a series of rationales provided by forecasters providing probabilistic forecasts to the question 'Will Russia successfully launch a moon mission on or before 1 Sep 2023?' Please summarize these rationales." (and then we pasted a few dozen anonymized rationales)
Out came an ordered list:
Not bad!
Then we tried to do what we essentially do now in our reporting with the following prompt: "The following is a series of rationales provided by forecasters providing probabilistic forecasts to the question “Will Russia successfully launch a moon mission on or before 1 Sep 2023?” Please provide two summaries - one summary with arguments in favor of success and one summary with arguments against."
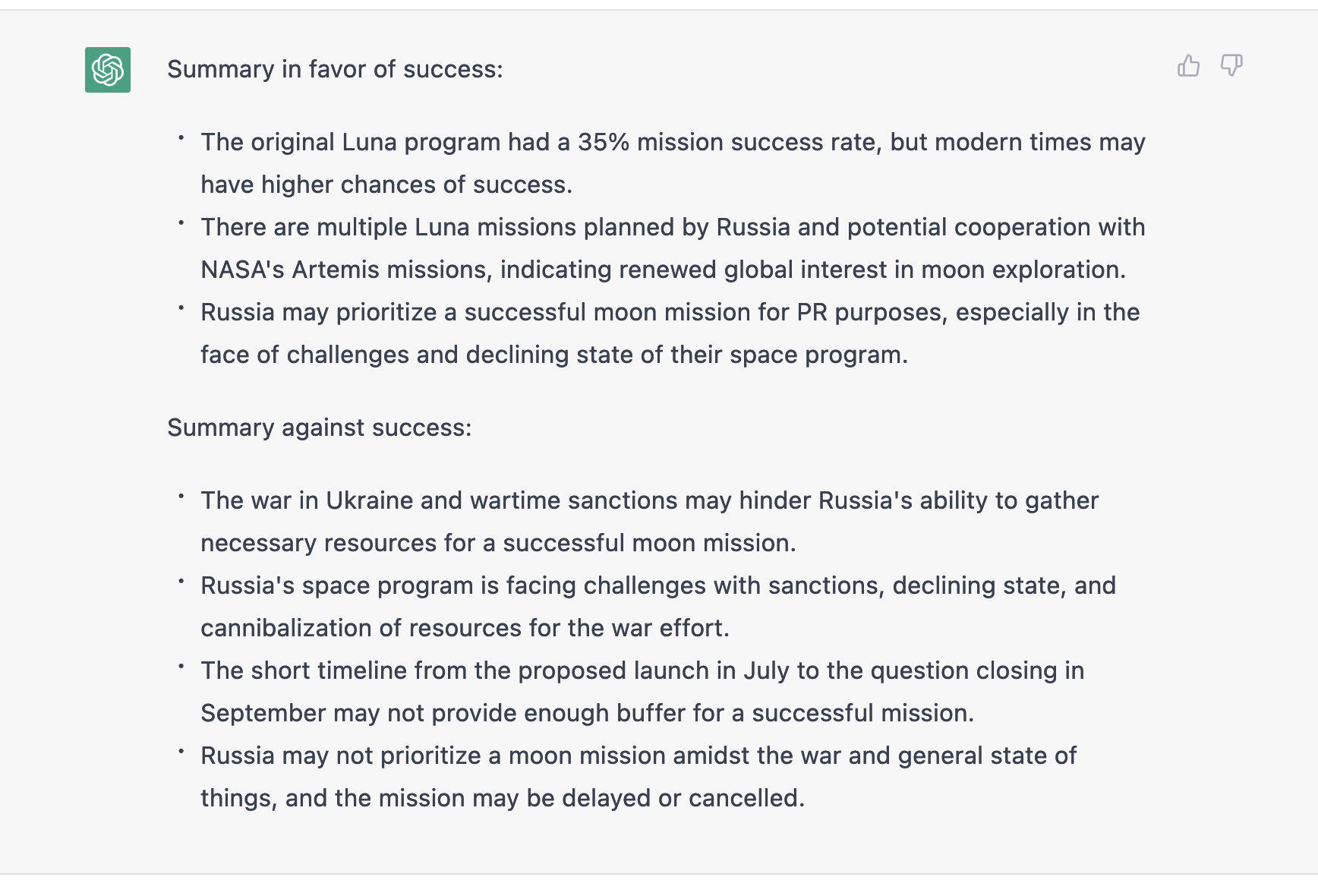
Again, really impressive!
With some capabilities we already have on our end, we could leverage this capability further in our reporting. For example:
- We use a simple Flesch-Kincaid scale to assess rationale quality. We could feed ChatGPT only our "best" rationales;
- We could group users by cohort and now quickly compare the rationales of different cohorts; and
- We could compare newer vs. older rationales to see what has evolved.
One fear we've had is similar to the problem many have with ChatGPT with other types of queries. Answers are presented as authoritative, but how do we know it's actually correct? How do we know this summarization is actually accurate, or that bias hasn't been introduced in the computation?
Given the nature of our work and the magnitude of decisions made based on the forecasts we're generating, we'll likely continue to keep a "human in the loop" as we produce our reports. However even using ChatGPT's summarization capability as a starting point is a huge productivity boost that allows us to scale much more than we could without hiring additional analysts.